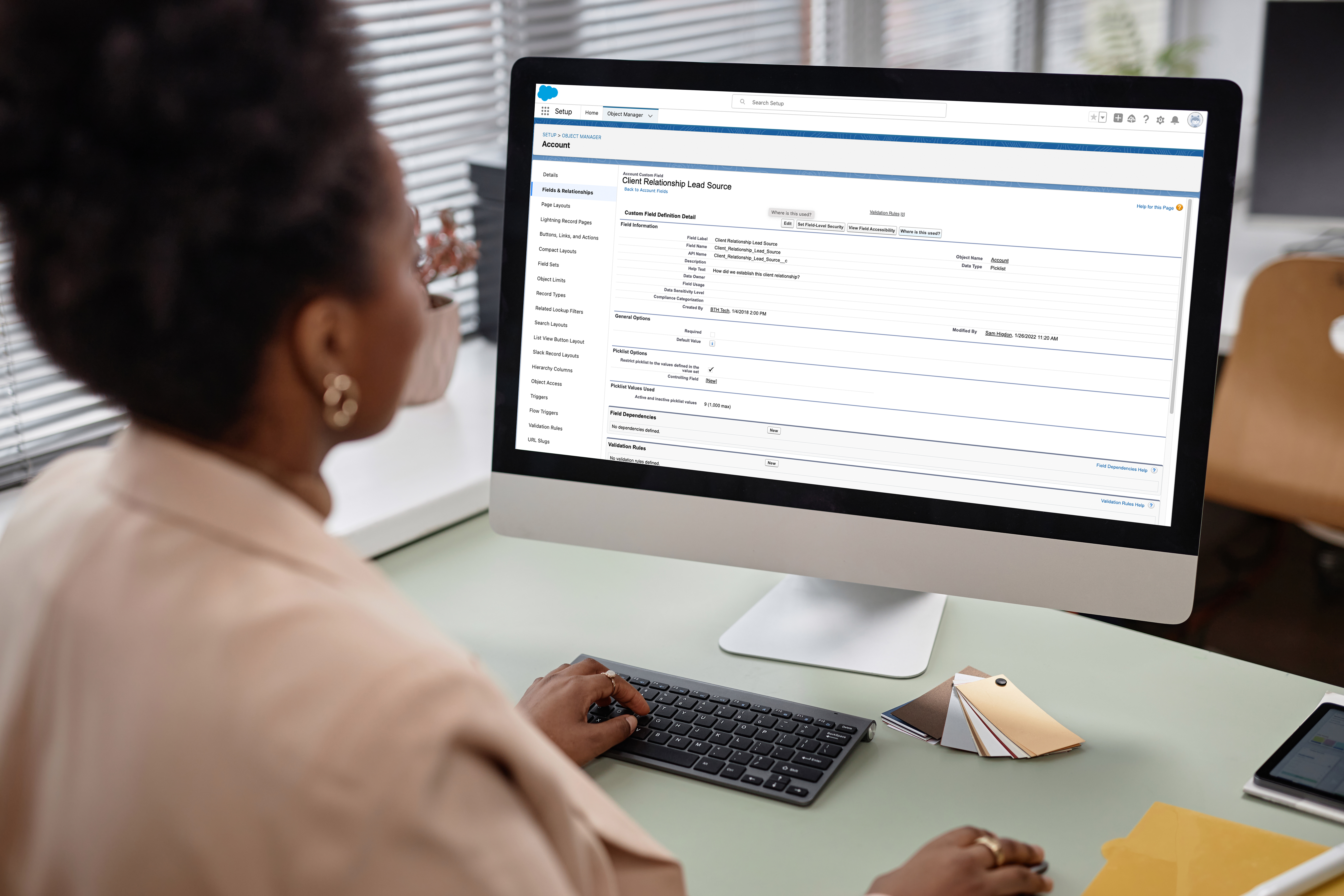
In today’s data-driven landscape, the importance of having reliable and clean data cannot be overstated. With high quality data, there are a variety of ways organizations can use their information to grow fundraising and have greater impact. Organizations are inundated with data from various sources, making it crucial to ensure that this information is accurate, reliable, and actionable.
However, many organizations struggle with this process or find other priorities competing for the time and resources it would take to analyze and clean their data. By prioritizing data cleaning and analysis, businesses can foster trust, enhance reporting, build a culture of data integrity, and streamline decision-making processes—all while preparing for future projects, including AI initiatives.
Why Clean Data Matters
Builds Trust Within Your Organization
Accurate and reliable data fosters trust across teams and departments. When stakeholders know the data they’re using is correct, they can confidently make informed decisions. On the other hand, poor data quality can lead to misinformation, decreased confidence in data-driven initiatives, and ultimately, resistance to relying on data altogether. Empowering teams to take ownership of their data can improve adoption of good data practices and confidence in the integrity of the data and how it’s used.
Improves Reporting and Analytics
Clean data leads to better reporting and analytics outcomes. By eliminating errors, reports can reflect true performance metrics, identify trends accurately, and inform strategic initiatives. This clarity is essential for guiding the direction of your organization and aligning teams toward common goals.
Data-Informed Decision Process
A culture that emphasizes data integrity creates an environment where decisions are based on solid evidence rather than guesswork. Clean data allows organizations to respond quickly to business process changes, understand customer needs better, and allocate resources more effectively.
Preparation for Future Projects
As organizations and industries evolve, embracing new technologies and methodologies, including AI and machine learning becomes essential. Clean, well-structured data is vital for these types of projects, as algorithms depend on high-quality input to generate reliable outputs. Investing time in data cleaning today will pay dividends both now and in the future.
Steps for Cleaning Your Data
Define a “Data Score” Process
A “Data Score” process can help prioritize data cleaning efforts. For instance, take a contact record and define key fields such as name, email, phone number, and address. Assign a weight to each field based on its importance—perhaps email is weighted more heavily than a secondary phone number. Then, calculate a score based on how many of these fields are populated or blank, allowing you to identify records that need immediate attention.
Review Picklist Values
Analyzing picklist values for accuracy and relevance is another essential step. Outdated or incorrect picklist options can lead to confusion and inconsistencies in data entry. Regularly reviewing these values ensures that they align with current business needs.
Run Field Usage Analysis
Use tools to assess which fields are frequently used, or regularly left blank, across records. This analysis can highlight fields that may be redundant, allowing you to streamline your data structure and enhance usability. This is particularly useful when preparing to migrate data, or when reviewing previously migrated data, to determine which fields are actually necessary or still useful.
Perform Bulk Updates for Consistency
Once you’ve identified areas to clean, many data cleansing actions can be performed by bulk updates to improve data consistency, formatting, and consolidation of fields. For example, standardizing the format of phone numbers or merging duplicate records can significantly enhance data quality across your database.
Introducing Data Governance
To build on your data cleaning efforts, it’s essential to implement a Data Governance process. This will help ensure ongoing data quality and compliance, laying the groundwork for future data initiatives and making sure the work you do now on cleaning data doesn’t go to waste. Ideally your data cleansing project was undertaken with the support of a Data Governance group. But if you started cleaning your data before your governance structure was in place, be sure to follow through on establishing Data Governance to ensure long term data quality.
Purpose of Data Governance
Data Governance is designed to manage data availability, usability, integrity, and security. It involves establishing policies and standards that guide data management practices across the organization.
Key Components of Data Governance
- Stakeholders: Identify who is responsible for data quality and oversight.
- Data Sources: Document where data is coming from and how it’s being used.
- Quality Standards: Set benchmarks for data accuracy and consistency.
- Consent and Privacy: Ensure compliance with regulations and protect sensitive information.
- Retention and Archiving: Define how long data should be kept and how it should be archived.
Next Steps
As you embark on your data cleaning journey, remember that maintaining high-quality data is an ongoing process. Implementing the steps outlined above will not only enhance the reliability of your data now but also strengthen your organization’s decision-making framework and prepare you for future technical innovation.
To continue your learning about data cleaning and governance, explore these resources:
- Salesforce Trailhead: Data Quality Module
- Data Management Best Practice Guide
- Data Governance Institute: Defining Organizational Structures
Need Help with Your Data Management?
We offer comprehensive data management services to support organizations in cleaning, analyzing, and structuring data for better decision-making and future growth. Contact us today to learn how we can help your data cleansing and governance journey.